Is now a good time to buy Nvidia?
Aaron Polhamus, CEO of Vest, analyzes the 24% drop in its stock and DeepSeek’s impact on its future. Opportunity or risk? Find out in this post:
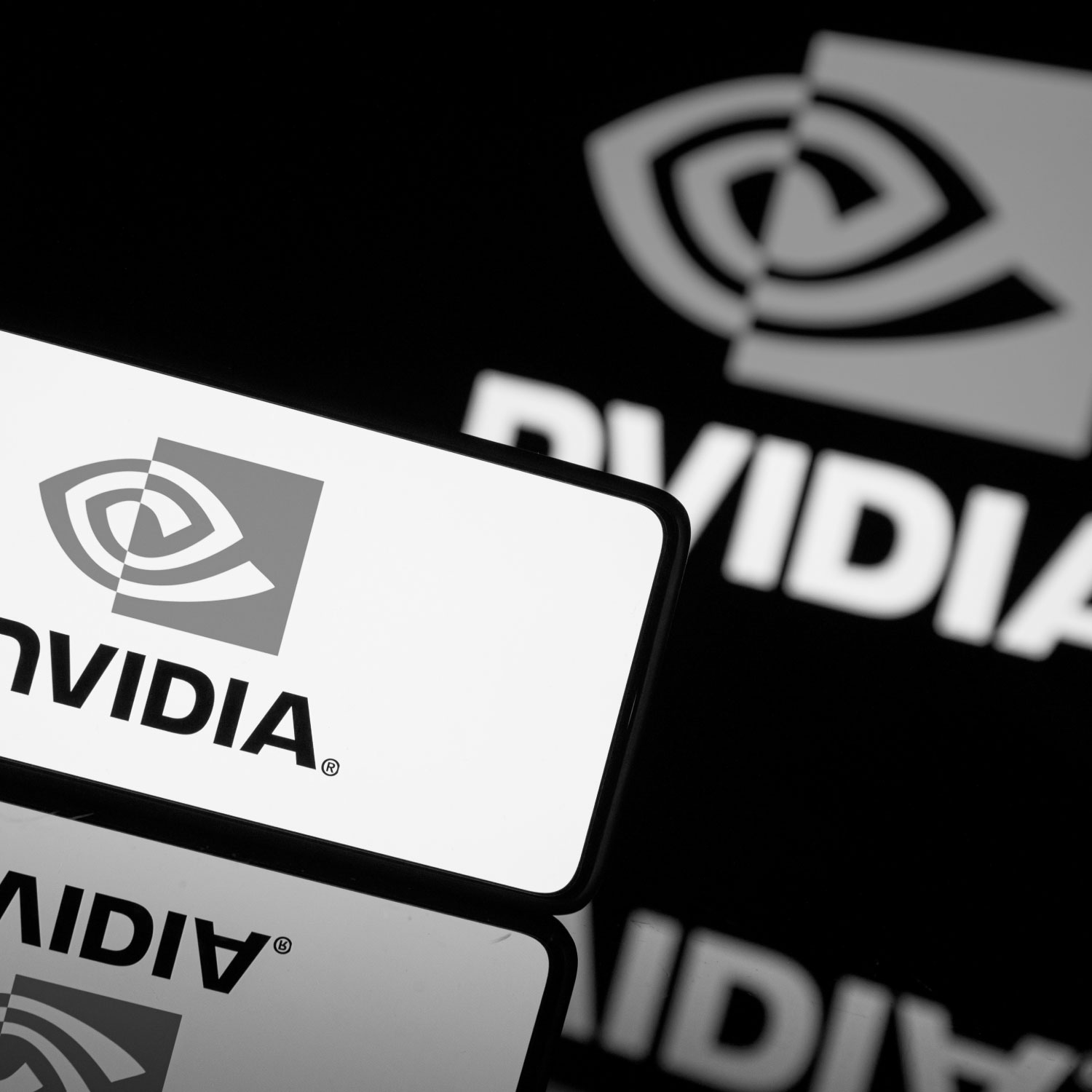
What follows is personal opinion and analysis, not investing advice, and does not represent the views of Vest or its officers. Read at your own risk.
Last week Nvidia stock shed almost 24%, throwing a bucket of ice water in the face of NVDA investors. The impact was broad: chip stocks, energy stocks, network technology providers — basically every industry that has been lifted by tailwinds from the AI gold rush — also experienced heavy selling pressure.
I work in the investments business, and friends started asking “Is now a good time to buy Nvidia?” After researching this question at length, in my personal investments the answer is “yes.” It’s true that DeepSeek represents the inevitable process of innovative new technology becoming more efficient and accessible. As this happens at an industry level, Nvidia’s market share and margins will come under pressure. The said, the company has plenty of room to run for three reasons:
If anything, we continue to underestimate the future demand for compute
Nvidia can mitigate its competitive vulnerabilities through M&A
We are still figuring out what actually happened: DeepSeek may not be accurately describing its true costs
A quick note about me: I am not an AI industry leader, and I will not be saying anything smarter about AI’s transformative potential here than Mark Zuckerberg¹, Sam Altman², or Dario Amodei³. I’m also not going to get nearly as technical as Jeffrey Emmanuel in his incredible write-up exploring the short case for NVDA⁴.
I am a career data scientist who’s been in the field since getting my MSc in Applied Statistics in 2009. I am also the CEO of Vest, a global investing platform focused on non-American retail investors. We bridge the gap between capital supply — hard-working people around the world — and capital demand — the global innovators who list on U.S. exchanges — and in the process help our customers find financial freedom through compounding asset growth in USD.
Like so many others, we’re fascinated by AI’s potential, and are scrambling to figure out what it means for our business and our clients. At the same time, our clients are trying to figure out what all this means for their portfolios. In my own personal investments I’ve been bullish on the space for a awhile, and was fortunate to be at the right place at the right time with my bets on chip stocks as AI took off:
It’s fun when convictions become reality
What happened
The crash came when DeepSeek publicly demonstrated a chain-of-through reasoning model that met or exceeded the performance benchmarks of US market leaders’ models for about 2% of the training cost and with significantly less computational power.⁵ This undermined the thesis that building smarter models requires ongoing multibillion-dollar investment in NVDA chips with their 90% gross margins. All of a sudden, it seemed like Nvidia’s future growth narrative collapsed and investors panicked.
Hype cycles come and go, but over the long-term valuations follow cash flows, and for a couple years now NVDA has been a cash gusher. Its recent and historical earnings beats have just been ridiculous:
Credit: https://finance.yahoo.com/quote/NVDA/analysis/
Credit: https://www.investopedia.com/nvidia-earnings-4775455
Big Tech is making truly remarkable future capital commitments to foundation model training and infrastructure: as I read the Stargate announcement, I remembered when I only ever saw numbers as big as $500,000,000,000 in the U.S. national budget.⁶ Nvidia’s current market dominance combined with future spending forecasts is what has powered its valuation. This is not 1999 dot com-variety hype: something very real and profound is happening.⁷ The question has always been who the winners and losers will be in this transformation. Since last week, that includes whether NVDA will continue to be one of them.
(Quick aside: we’ll talk about “training” versus “inference” below. “Training” is when an AI model is actually built by exposing it to terabytes of data. This is where Nvidia’s chips shine. “Inference” is the process of asking a model questions and getting answers after it’s trained, and it involves solving slightly different problems.)
DeepSeek claims to have trained its R1 chain-of-thought model for $6M, compared to costs of over $100M at Anthropic and OpenAI. Just as importantly, companies like Groq and Cerberas are running inference calls on dedicated, non-NVDA hardware with up to 57x the efficiency of GPU-based inference.⁸ It’s a big deal, and Jeff’s analysis does a masterful job unpacking how multiple competitive threats are conspiring to undermine both NVDA’s margins and market share going forward.⁴
The bull case for Nvidia: AI is growing faster than any of us could have imagined, and Nvidia is the market leader.
The bear case: DeepSeek just broke the market, compressing margins and unleashing Nvidia’s competition.
Business leaders immediately invoked Jevons paradox, with Satya Nadella writing:⁹
“Jevons paradox strikes again! As AI gets more efficient and accessible, we will see its use skyrocket, turning it into a commodity we just can’t get enough of.”
In other words, what Big Tech is about to lose through margin compression, it is going to more than make up for in volume from widespread adoption.
The Economist argues that Big Tech’s leaders may be too sanguine about Jevons paradox in this case: in order to apply, the total increase in demand resulting from efficiency gains in the usage of a commodity have to more than offset the total cost savings resulting from the efficiency increase.¹⁰ It’s relatively rare that this happens — energy has been more the exception than the rule — and with only 5% of American businesses using AI, with only 7% reporting plans to do so in the future, it’s not inevitable that the paradox will apply here, either.
The key question for Nvidia investors is: As training and inference costs for AI continue to fall, will Jevons paradox apply, and if so, will Nvidia continue to capture a large enough share of the created value to grow into its current valuation? I believe that the answer is “yes,” and am a “buy” on NVDA for three reasons:
Nadella is right about Jevons paradox. I believe that if anything we are underestimating future demand for compute
Nvidia is the industry’s 1,000 pound gorilla: if they can’t vanquish their competition on the field of battle, they’ll buy them out
DeepSeek may not be telling the whole story: industry insiders speculate that DeepSeek leveraged U.S. foundation models in its training while downplaying its true costs
Exponential growth of compute
Consider this plot from The Singularity is Near, showing the exponential growth in the number of calculations that can be performed per second for $1,000 since the advent of digital computing. Kurtzweil published the book in 2005, and the red arrow is my own annotation drawing a line to the present day. At approximately 10¹⁶ calculations / second, a computer approximately matches the computational capabilities of the human brain:
Source: https://www.singularity.com/charts/page70.html
Kurtzweil’s accuracy has been uncanny: Open AI’s o3 model, which achieves genius-level human performance at solving hard math problems, costs as much as $3,000 per query.¹¹ But remember, DeepSeek / Cerberas / Groq just cut inference costs — the cost of asking questions — by 45x. We’re about to blow past the “$1,000 to pay a computer to be as smart as a human” benchmark if we haven’t already.
Pundits talk about how high this $3,000 cost is, and how it illustrates the challenges to the unit economics of foundation model creators. Sure, but they miss the point: the fact that we are even arguing at all about whether $3,000 is a reasonable price to charge to consult a computer that’s smarter than a math PhD is a wild paradigm shift. Can you even imagine having that conversation in early 2020? Today, it’s normal. This will cost a lot less by the end of the year. Much, much less in 2027. Before too long, we’ll be running super powerful inference servers on personal laptops.
At the same time, global energy consumption dedicated to compute is increasing exponentially. As a civilization, we are consuming exponentially more energy to run computers that are becoming exponentially more intelligent. We are seeing exponential growth within exponential growth of global compute. Increasing efficiency makes this possible. Seen from this perspective, DeepSeek’s breakthrough wasn’t surprising at all: it was inevitable.
So the future of the compute industry is bright. However, DeepSeek just showed us how Nvidia’s market share will contract and their margins will shrink. It’s likely that in the future Nvidia won’t continue to be used in 97% of all AI learning systems while enjoying 90% margins.¹² Beyond DeepSeek, companies like tiny corp are working furiously to “commoditize the petaflop,” writing CUDA-like software for AMD GPUs that deliver more efficient per-dollar compute.¹³ Without a doubt, the competition is here.
As professor Scott Galloway notes, “eventually everything goes Walmart/Tiffany’s.”¹⁴ Premium, high-cost, cutting edge models are the “Tiffany’s” option in this example, and Nvidia is still the unquestioned provider of Tiffany’s-grade GPUs. While premium LLMs may become less dominant as a share of the overall market, the exponential growth dynamics of the industry mean that Nvidia likely has much more room to run. Furthermore, Apple proves that it is possible to offer a premium product at massive scale with high margins for decades. The key for Nvidia going forward will be to maintain their premium performance advantage while solving for vulnerabilities related to efficiency.
Defense through M&A
Facebook dominated social media in the early 2000’s, but was late to the party on mobile social and messaging. So they bought Instagram and WhatsApp, consolidating a huge chunk of the global social media ecosystem. Seeing the rise of streaming, Google acquired YouTube for $1.65Bn in 2006. Today, these three acquired platforms are the most popular social networks on the internet after Facebook. Meta and Alphabet wrote the playbook for aggressive M&A as a growth strategy for Big Tech:
Source: https://www.shopify.com/blog/most-popular-social-media-platforms
Nvidia is not going to sit on top of its $38.48Bn cash pile being sad while the competition laps it. It’s either going to increase the efficiency of its own chips, or it’s going to start buying out upstarts and folding them into its hardware / software ecosystem. Cerberas’ valuation range, for example, runs between $1B and $10Bn.¹⁵ I predict that Nvidia will make some major acquisitions in the coming year, starting with inference, where they are apparently the weakest. This dynamic already played out with networking technology in 2020 when Nvidia acquired Mellanox, unlocking the build-outs of the massive GPU clusters that power LLM training today.¹⁶
Dirty deeds, done dirt cheap (allegedly)
A Chinese company committing IP theft to create a low-cost knock-off of a world class American product is a story as old as the U.S.-China trading relationship. To be clear, I am not jumping on that train here, and even if DeepSeek does turn out to have “Chinese knock-off” dynamics it would still be an extraordinarily impressive engineering accomplishment. Hats off to DeepSeek’s incredibly badass developers 🎩
That said, questions have been raised over the past week about whether DeepSeek’s official version of their story matches reality:
OpenAI claims that the DeepSeek team used “distillation,” the process of recycling calls and responses against another LLM in model training.¹⁷ ¹⁸ If so, this would be a gross violation of their SLA. It would also affirm that high-cost, NVDA-powered training runs are still required to push AI’s intelligence frontier.
Experts are heavily scrutinizing DeepSeek’s claim to have spent $6M on model training, as well as the number of NVDA GPUs used in their server cluster. By one estimate, the company spent up to $1.6Bn on building its cluster, including 50,000 NVDA GPUs.¹⁹
DeepSeek’s achievements are impressive and it clearly found some efficiencies in the incredibly expensive model training process. Whether it “broke the market,” however, is a question that will be debated in the coming weeks. Today, there are grounds for healthy skepticism.
In conclusion
Nvidia today is the monopoly supplier of the hardware and software required for the training of cutting edge AI models in an industry whose exponential future growth will likely exceed the forecasts of Wall Street analysts. While its margins and market share will contract as the industry evolves, it will defend its dominance in training with ongoing innovation while shoring up its weaknesses in inference through M&A, which it already has a track record of doing successfully. Finally, the extent to which DeepSeek truly broke the unit economics of the AI industry remains to be seen. Experts will continue to question the true scale of their server cluster and investigate the possibility that premium models — trained on massive Nvidia GPU clusters — were distilled during the model training process.
The P/E ratio today of the S&P500 is 30.18²⁰, while Nvidia’s P/E ratio is 47.39²¹, a ~50% premium over the broader market. Given the company’s prospects, this does not strike me as unreasonable. I’ll be trimming some of my positions in the coming days to increase my NVDA exposure.
I think that the creators of foundation models — OpenAI, Anthropic, etc. — have much more to be concerned with, as the DeepSeek / Groq / Cerberas combination represents their direct competition. Nvidia, on the other hand, sells shovels to a gold rush that, like the internet, will never let up.
It’s an incredibly complex ecosystem, and calling winners and losers is a hard game. In a 1999 speech at Sun Valley, speaking to a crowd that was exuberant about the bull run in dotcom stocks, Warren Buffet issued a note of caution:²²
All told, there appear to have been at least 2,000 car makers in an industry that had an incredible impact on people’s lives. If you had foreseen in the early days of cars how this industry would develop, you would have said ‘Here is the road to riches,’ [yet,] after corporate carnage that never let up, we came down to three U.S. car companies — themselves no lollapaloozas for investors.
In any transformative technological revolution, the ultimate winners and losers are often not the first movers. Investors who deploy billions of dollars push the innovation frontier forward, but there are no guaranteed paydays. That’s why it’s called “venture capital.” In addition to modestly increasing my position in NVDA, I’ll also be exploring ETFs that provide sector exposure to energy and compute, allowing me to bet on the trends discussed here without over-concentrating on a single company.
Lots of opportunity and peril out there. Develop and hone your own convictions, keep a level head, and never invest more on high-risk positions than you’re able to lose while still sleeping well at night. Next post I’ll zoom out and speculate on what AI means for business in general and investing in particular.
For illustrative purposes only. Does not represent an investment recommendation. For more information, please see our Social Media Disclosure.
References
https://about.fb.com/news/2024/07/open-source-ai-is-the-path-forward/
https://youtubetranscriptoptimizer.com/blog/05_the_short_case_for_nvda
(Of course something very profound was happening in 1999, too, as the internet began to seriously disrupt traditional business models. However the run-out on a price-to-earnings basis was far more exaggerated then, with the Nasdaq hitting a PE ratio over 200, compared to about 49.31 today (see https://fullratio.com/stocks/nasdaq-ndaq/pe-ratio). Prices are rich in the AI boom, but they are supported by cash flows in a way that dotcom companies were not.)
https://www.investing.com/academy/statistics/nvidia-facts-and-statistics/
https://geohot.github.io/blog/jekyll/update/2023/05/24/the-tiny-corp-raised-5M.html
https://nymag.com/intelligencer/article/deepseek-may-be-the-walmart-of-ai.html
https://www.crunchbase.com/organization/cerebras-systems/company_financials
https://www.theverge.com/news/601195/openai-evidence-deepseek-distillation-ai-data
https://www.berkshirehathaway.com/1999ar/FortuneMagazine.pdf